[[{“value”:”
Language model development seems to have hit a ceiling. OpenAI’s upcoming Orion model barely outperforms GPT-4, its predecessor, according to a new report. This slowdown affects the entire AI industry.
The Information reports that OpenAI’s next major language model, codenamed “Orion,” delivers much smaller performance gains than expected. The quality improvement between GPT-4 and Orion is notably less significant than what we saw between GPT-3 and GPT-4.
What’s more, Orion doesn’t consistently beat its predecessor in areas like programming, showing improvements only in language capabilities, according to The Information’s sources. The model could also cost more to run in data centers than previous versions.
Running out of training material
OpenAI researchers point to insufficient high-quality training data as one reason for the slowdown. Most publicly available texts and data have already been used. In response, OpenAI created a “Foundations Team” led by Nick Ryder, The Information reports.
This aligns with CEO Sam Altman’s statement in June that while data exists in sufficient quantities, the focus will shift to learning more from less data. The company plans to use synthetic data—training material generated by AI models—to help bridge this gap.
The Information notes that Orion has already trained partially on synthetic data from GPT-4 and OpenAI’s new “reasoning” model o1. However, this approach runs the risk of the new model simply “resembling those older models in certain aspects,” according to an OpenAI employee.
LLM stagnation poses a challenge for the industry
The slowdown in LLM progress goes beyond OpenAI. The Verge recently reported that Google’s upcoming Gemini 2.0 is falling short of internal targets. Anthropic is rumored to have halted development on version 3.5 of its flagship Opus, releasing an improved Sonnet instead – possibly to avoid disappointing users and investors.
Open-source models catching up to billion-dollar proprietary ones over the past 18 months further shows this industry-wide plateau. This progress would be unlikely if major tech companies could effectively convert their massive investments into better AI performance.
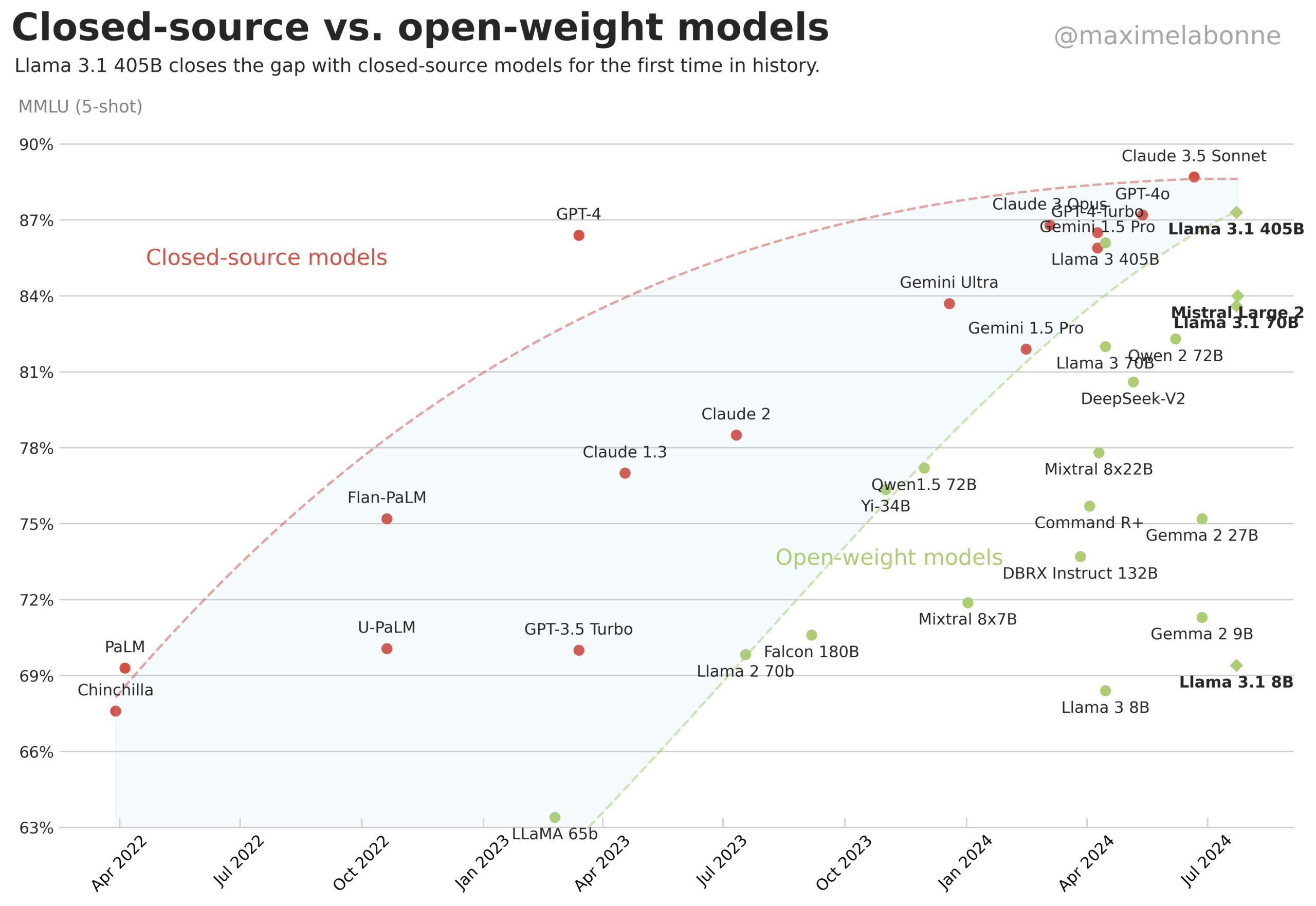
But in a recent interview, OpenAI CEO Sam Altman remained optimistic. He said that the path to artificial general intelligence (AGI) is clear, and that what is needed is a creative use of existing models. Altman could be referring to the combination of LLMs with reasoning approaches such as o1 and agentic AI.
Noam Brown, a prominent AI developer at OpenAI and former Meta employee who helped create o1, says Altman’s statement reflects the views of most OpenAI researchers.
The new o1 model aims to create fresh scaling opportunities. It shifts focus from training to inference—the computing time AI models have to complete tasks. Brown believes this approach is a “new dimension for scaling.”
But it will require billions of dollars and significant energy use. This is a key industry question for the coming months: Does building ever-more-powerful AI models—and the massive data centers they need—make economic and environmental sense? OpenAI seems to think so.
Gemini-LLM in AlphaProof was “basically cosmetic”
Google AI expert François Chollet criticized scaling language models for mathematical tasks. He called it “especially obtuse” to cite progress in mathematical benchmarks as AGI proof.
Chollet argues empirical data shows deep learning and large language models can’t solve math problems independently. Instead, they need discrete search methods—systematic approaches that check various solution paths rather than predicting likely answers like language models do.
He also criticized using “LLM” as a marketing term for all current AI advances, even when unrelated to language models. He pointed to Gemini’s integration into Google Deepmind’s AlphaProof as “basically cosmetic and for marketing purposes.”
“}]]